
Cyquential AI
Marrying the reasoning capabilities of Traditional AI with the generalization power of Modern AI
In Artificial Intelligence, there exists two parigrams: Furthermore, both appear to solve the problem the other struggles with. This begs the question: What if there was a way to combine both technologies?
Meet Cyquential AI. This is accomplished through the self building architec ture, where neurons and synapses (forming of the repetition) form by themselves as opposed to being fixed hyperparam eters.
Features
Independent Continual Learning, the human way
Cyquential AI learn without needing any labeled data or even a teaching signal. This means that learning can be done without needing to create any type of error function or ”correct answer”, meaning that involvement with the AI is very hands free. This is accomplished through the self building architecture, where neurons and synapses form by themselves as opposed to being fixed hyperparameters. This also enables Cyquential AI can perform full continual learning, with very minimal catastrophic forgetting as no neurons are deleted or changed. No retraining, weight freezing, or any manual involvement is required when adding new data or tasks to learn. The learned representations are still distributed as in a neural network architecture, which creates preservation of all the useful capabilities that modern deep learning yields.
Inductive logic and few shot generalization with no black box
Describe the item and include any relevant details. Click to edit the text.
Very Low Compute Power, Easy Portability
Because Cyquential AI only activates nodes that are relevant to the current task at hand, and does not require computations through the entire network, the overall processing power Cyquential AI consumes is very low. The size of the network dynamic, and so knowledge can even be trimmed to isolate core tasks; additionally, nodes can utilize all previous information, so additional learned items take up minimal space.
Formal Abstract
Contemporary artificial neural networks and classical AI both suffer from the symbol binding and grounding problem, an issue that prevents distributed representations of perceptual knowledge from internal object binding. Cyquential AI is a new type of symbolic model designed to subvert this problem. It uses a new propositional learning hypothesis, called the Cyquential hypothesis, that aims to re-engineer physical symbol systems in such a way that symbols are generated autonomously in the knowledge base from real time perceptual data, as opposed to being hardcoded in. The symbols generated often represent task generalized object representations, and are created sequentially such that they build off each other. In addition, the algorithmically generated labeled symbols are stored in a continually growing, flexible network-like structure. This means continual learning only takes few to one shot for different tasks, and knowledge of how to do the previous task is never forgotten, even when adding new tasks. It is found that the Cyquential-built AI can adequately perform one pass-through few shot recognition on the MNIST fashion dataset (65 percent accuracy), while using no deep learning or any external data. In addition, it performs continual learning to recognize MNIST handwritten digits, while still being able to recognize MNIST fashion simultaneously.
2022 Ohio State Undergraduate Cognitive Science Fest: Poster 1st Place
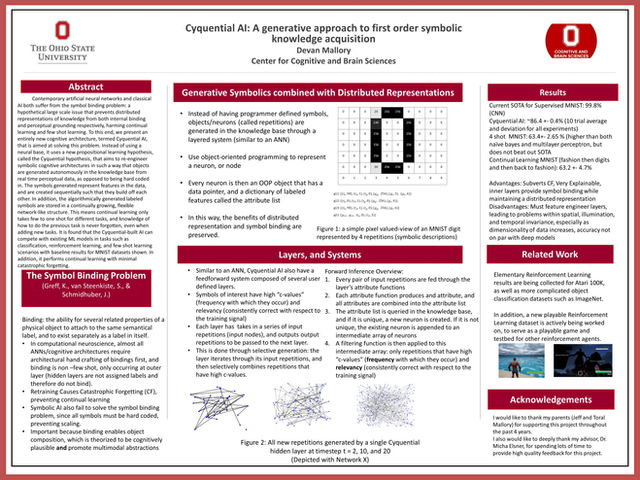